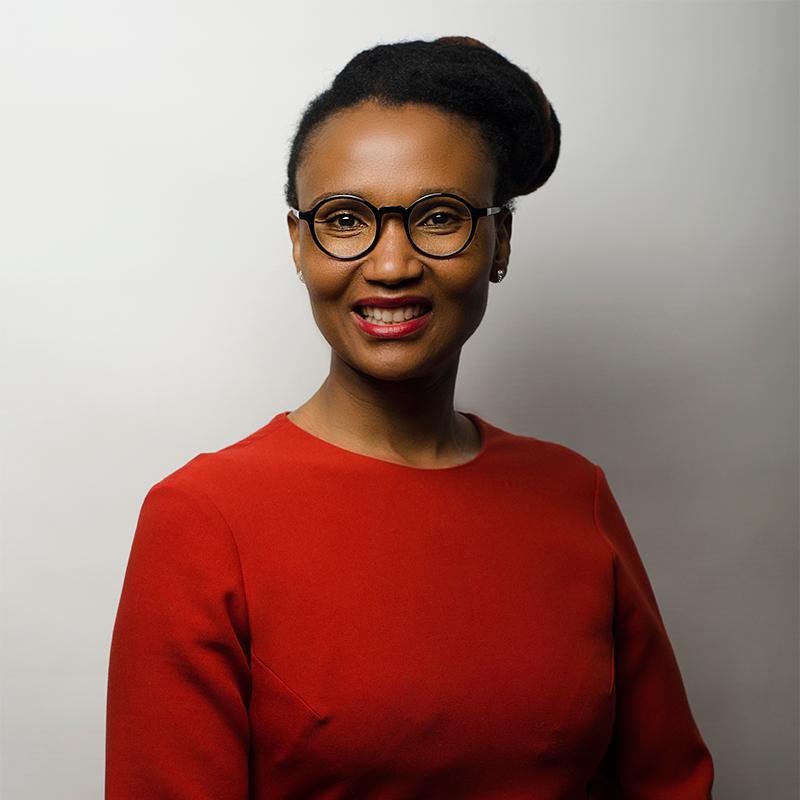
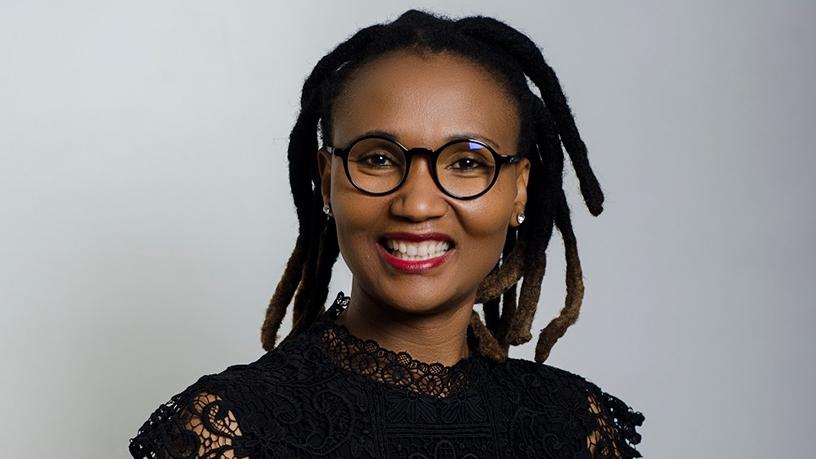
Data modelling has been around for many years within the information management discipline. As stated by SAP, "data models are the foundation for data exploration and visualisation".
I believe it is certainly an important competency for any business intelligence (BI) or analytics centre of excellence, contributing to the deploying of data and analytics systems that deliver true business value.
Data modelling, as defined by DAMA, is "the act of creating a data model (conceptual, then logical and finally physical), and includes determining the data needs of an organisation, and its goals".
As part of this journey of creating a data model, experienced data modellers deploy specialised techniques like data profiling which assists in exploring existing datasets to detect patterns or anomalies in data.
Furthermore, the design of the data model assists in enabling information users to answer business questions by discovering, analysing and structuring the data elements into the "where", "what", "when" and "how" insights about a business event or customer lifecycle that is hidden in the raw data but is often difficult to find.
Ultimately, a comprehensive data model needs to structure data in a granular level for both internal and external data captured to effectively represent or depict a real-life scenario in the context of the business operations.
Keys to data modelling success
Data analysis and process-driven design approaches are key to the success of utilising the data modelling function as a fundamental driver for analytics success.
Data modelling brings and holds concepts together; where things don't make sense, the data model serves as a point of reference; and therefore, the data modelling function requires seasoned data experts that are also excellent communicators.
In other words, a strong data modeller must be able to start business conversations, facilitate discussions and involve business representatives continuously while seeking to get deep understanding of (1) the business subject areas and processes; (2) the business systems and the data it produces to assimilate into new information; as well as (3) the business questions that decision-makers require answers to.
Furthermore, it is important for a data modeller to understand how the data model will be used and where it is going to be used, especially for big data, as there are many ways to model the data.
A data model is not complete if the "fit-to-purpose" scorecard is not defined.
A well-defined data model plays a significant role in the governance of data assets, especially to clearly classify data elements where the classification drives data security requirements in alignment with regulatory standards. This implies that a properly defined data model also provides business and technical metadata, as well as governance metadata like the ownership/stewardship allocation per data subject.
Rich metadata like this will allow the technical team to address security classification before continuing with any development work in the analytics systems. In a case where the data needs to be pulled from source, it is important to understand the security requirements from the data owners, including what data needs to be accessed, how, and who needs to have access to the data from source into BI or analytics layers.
All of this must be captured in the data model to guide the development. Data insights will want to identify any impacts to their data structures and changes that may be needed to enable compliance.
How do I know that a model is good?
The quality of a data model needs to be checked and assessed based on the pre-defined dimensions. A data model is not complete if the "fit-to-purpose" scorecard is not defined.
A typical "fit-for-purpose" scorecard includes the following factors, among others:
The model coverage: It has a significant impact on quality and usage of data modelling. Scope should be explicitly stated.
Ease of understanding: This is a prerequisite to proper use of the model by different users.
Non-redundancy: The importance of data non-redundancy is largely determined by the relative importance of update and enquiry transactions, together with performance requirements.
Definition of business rules and security requirements: The more rules that are defined in the model, the better, as the model serves as a point of reference for all data-related development. This will ensure the data asset is well classified in the foundation level.
Stability and flexibility: It is important that the model should have the ability to accommodate business change with less impact modification. Flexibility relates to ease of modification.
Performance: Adequate performance needs to be achieved without impacting the logical structures, or that any such impact will be minimal. Data models need to be tested and validated to check how long the queries take to run.
Ultimately, data modelling relieves stress throughout the data and analytics systems development lifecycle, from supporting the business analysts to clearly articulate business requirements, clearly articulating rules and objectives for developers and data engineers if the data model is properly defined and validated, and finally structuring the data elements in a way that makes it intuitive for analytical end-users to access, analyse and generate insights from the data.
It can significantly reduce end-to-end development work, meaning the business questions and business value can be realised much quicker, especially if the data modellers involve the business stakeholders effectively.
With the data model being so fundamental in the success of BI and analytics systems, it is no wonder that we observe a significant increase in organisations reviewing and revamping their data modelling and data design practices.
Share