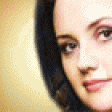
It has never been more important for business to make use of data-driven insights to formulate actionable strategies, but finding quick, intuitive, simple ways to convey critical insights and concepts has assumed an equally important role. Businesses can utilise data visualisation for their broader team to consume mass amounts of data and make informed decisions faster.
So says Melissa Jantjies, senior associate systems engineer at SAS, adding that not everyone can be a data specialist who develops BI reports. Fortunately, she says, we’re moving into a space where analytics are becoming more platform-driven and companies can more readily adopt this into their strategies. From a data visualisation perspective, platform-driven analytics can suggest presentation options based on the data available, for example presenting information on a map overlay to represent information geographically. This can help users identify gaps in the business and opportunities to fill those gaps.
Paul Morgan, business unit lead for Data, Planning, and Analytics at Altron Karabina, says data can also be used to understand poor performance in certain areas of the business. He cites examples including operational efficiencies, or whether the business has cash-collection issues, or if poor sales performance now is going to impact the business in three months’ time.
“You can manage the risk and protect the viability and sustainability of the business by using data. In addition, with advanced analytics, employee and customer churn, or plant downtime, can be predicted, and data can assist with improving the efficiencies and allow business to do them better. Finally, you can leverage data to do things differently, such as identifying new products, new geographies or even new approaches to business. For example, General Electric famously used IoT and sensor data and analytics to turn its electric turbine sales business into a ‘Power-as-a-Service’ solution that guaranteed power delivery,” says Morgan.
It has been proven repeatedly that understanding the business and its client base allows it to progress and adapt to the ever-changing landscape, adds Archana Arakkal, machine learning engineer at Synthesis Technologies. “Truly understanding your customer by using information that you already have is exactly where data can be turned into a business advantage. An example of this is personal accounts; should a person discover they’re spending way above their income, then they’re able to now make an informed decision regarding their future actions. The alternative is an individual who has no data trail of their expenditure and hence has no visibility of their assets; they’re likely heading towards bankruptcy. The example was based on an individual but can be used in the same context for businesses having data points that can be understood and then truly allow for future business prospects,; allowing your business to become more client-centric and understanding their real needs.”
Gaining insight
“Effectively analysing data can produce insights the business did not previously hold,” adds Andreas Bartsch, head of Service Delivery at PBT Group. “Such insights can be critical to a business’ future planning, key decisions, and overall sustainability and success. But more than that, these insights can be leveraged to gain a clearer understanding of the customer base and develop solutions targeting them in more innovative ways. While there are a variety of business processes, analytics models, governance and other factors that contribute to the value of data analytics, the key to reaping such benefits does not lie in the analytics alone, but also in the quality of the data being analysed. However, to fully unlock the business advantage of data, its integrity must be without question. For instance, in the case of customer analytics, nobody wants to be contacted about a possible offering that doesn’t meet a current need, or worse, about an offering they already use from that same provider. Therefore, it’s critical for data analytics to help deliver the required competitive advantage. Investing in analytics without examining data quality, however, becomes meaningless and will not garner the desired returns the company was expecting when using data to the advantage of a business.”
What are the key areas enterprises need to address when building an effective data strategy? Jantjies says for an enterprise to become a successful data-driven business, it needs to be able to foster a collaborative, goal-oriented culture. “An effective data management strategy spans the entire analytics lifecycle. Data is accessible and usable by multiple people – data engineers and data scientists, business analysts and less technical business users.”
If the basics of a data strategy or data foundation are not as effective as they should be, then no technology investment can help realise a business advantage.
Andreas Bartsch, PBT Group
Morgan adds that data needs to be dealt with in the same way as organisations manage all their valuable assets – they must create strategies to protect, optimise and leverage the asset. “When you protect a physical asset, like a mine or a plant, you need to ensure that unauthorised people can’t easily access it and damage or steal items. When figuring out how to protect your data asset, you need to focus on risk management themes such as data security, authentication, access control, disaster recovery and backups – there’s no point investing in an asset if its value is easily lost. To optimise a physical asset, you need to focus on making sure that all the parts are working efficiently, and that it has been set up in the best way. Similarly, when strategising about how to optimise a data asset, there are several critical themes to look at, such as how to ensure high performance, giving query results in seconds instead of hours. Also, how to deal with scaling users and data volumes, how to ensure value for money, and how to structure data so that it can be accessed from one location, whether virtualised or physical.
“Mechanisms are needed to consolidate data to gain a view of the whole data estate, and data must be easily accessible to users. Authentication and access control are necessary, but it shouldn’t take five minutes to log on. Organisations need to find an easy and secure way to access and use this data, which requires adoption and enablement processes to measure who is using it and ensuring they have the skills and training to take advantage of these platforms and, inherently, the data.”
Solid foundations
According to Bartsch, many companies may believe that their data foundations are running smoothly, which often results in them focusing on the technology trends and elements needed to build upon data strategies. “However, if the basics of a data strategy or data foundation are not as effective as they should be, then no technology investment can help realise a business advantage. A solid data strategy not only requires focus on technology investment, but more importantly, the current regulatory environment needs to be considered, as do data governance and security structures. This enables the company to ensure that the information at its disposal is accurately governed by controls and that a data security programme is in place.
“The second element entails building a reputable use case on which to test the data strategy. Making sure the whole data foundation can deliver on the required outputs of the entire organisation means that one or two key use case scenarios must be tested. Finally, a business should never neglect the roles of the people involved in the overall process. Data scientists ‘paint’ the picture, make the observations, and are the masters of getting the message across. For their part, data engineers hold the skills needed to build a solid data foundation. They are, therefore, responsible for building an enabling environment for the data scientists to capitalise on.”
When it comes to developing data protection, governance and redundancy policies, Bartsch says a critical step in this process is gaining an understanding of the regulations as relating to protection, governance, and redundancy policies. More specifically, an organisation must know the industry-specific nuances of each of these and how it applies to the business. “This will empower the company to build data management processes with all these compliance components in mind. The advent of technologies like artificial intelligence, machine learning, and the Internet of Things has resulted in what feels like an influx of data regulations that constantly change. If the organisation isn’t familiar with the governance aspects of each of these, then it faces significant risks, both financial and reputational. Compliance can be effectively achieved when it’s based on the principles of being responsible stewards of the data, being able to identify and classify data that is of a sensitive nature, and govern overarching principles linked to company policy around using data responsibly. Putting in place a company ethos around data management, guided by the above principles, the tone is set for effective data protection, governance, and redundancy to be achieved. If strong data management structures exist, based on solid principles, then compliance should naturally be achieved.”
It’ll all end in tiers
Data tiering is another essential component of the data strategy employed during the data engineering process, since it is the process whereby each data set is analysed and classified, says Arakkal. This data is then optimally placed into the most relevant storage device. This is part of the data strategy process of identifying the data classification needs to best suit the infrastructure. Since data is essential for a multi-functioning organisation to advance their opportunities, it’s important to only expose data that is of a high calibre.
Truly understanding your customer by using information that you already have is exactly where data can be turned into a business advantage.
Archana Arakkal, Synthesis Technologies
Speaking of optimising data ingestion, Arakkal says the initial stage of any data project is focused on data engineering, which incorporates, but is not limited to, data ingestion, data cleaning, data governance, data privacy and data redundancy.
“These various components can take an inordinate amount of time and need to be given the dedicated focus they require. It can take 60% to 80% of scheduled time to collect and clean data in any analytics project. As a result, it’s even more important to optimise data ingestion pipelines. The reality of the situation is that data scientists spend less time optimising algorithms and more time wrangling data in order to begin their analytics work and as the size of the data within an organisation grows, the time associated with this process also grows. To tackle this data ingestion optimisation problem, impose an effective data-driven strategies plan to accommodate the potential difficulties in an end-to-end system, and, as far as possible, automate data ingestion pipelines. Use AI to help isolate data anomalies, and remember that ingestion pipelines are to be built keeping the self-service model in mind. Leverage data governance policies to keep your data clean, and only expose cleansed data. Unfortunately, there is no single blueprint to assist with optimising data ingestion. It is, however, possible to plan effectively by understanding where the weak points lie in the end-to-end system and effectively draw mitigation strategies that may assist with the success of the project. This could include scheduling more time for data ingestion, assigning more people to assist, bringing in external expertise or deferring the start of developing the analytic engines until the data ingestion part of the project is well underway. While it’s effective to have a manual process for creating a data ingestion pipeline in the past, data has become far too large to do so in this manner.”
Rise of the machines
Augmented analytics, and the use of machine learning and AI are also being used to assist with data preparation, insight creation and insight description, to improve data discovery and interpretation of analytics. “It also augments the way that expert and citizen data scientists work by automating the creation, management and growth of many aspects of the data science, machine learning and AI model development, management and the deployment of those models,” says Jantjies.
Blockchain is also being used to help prevent fraud, but it’s not a fool-proof method against sloppy security and poor data practices. It does promise, however, to improve the security of transactions for people and ‘things’ in real-time. While the use of blockchain technologies is still in the early stages, it is actively being investigated as a new type of distributed data environment for many virtualised network systems applications. There are two categories of data related to blockchains, namely data at rest and data in motion.
Exporting the static blockchain data into an analytics platform allows users to review various transaction characteristics, segment transactions, analyse trends, predict future events, and identify relationships between the blockchain and other data sources. Making blockchain data available for analysis can be helpful for anti-money laundering, customer intelligence, fraud detection, revenue forecasting and new services creation. “With the advent of streaming analytics, blockchain data in motion offers additional opportunities for analysis, which can help identify, in near-real time, changes in the blockchain’s activities. Seeing these changes as they’re happening provides an opportunity to take immediate action to address activity in the blockchain as transactions are occurring. Moreover, analytic models developed using static data can be applied to the data in motion to ensure the integrity and authenticity of a blockchain,” concludes Jantjies.
Share