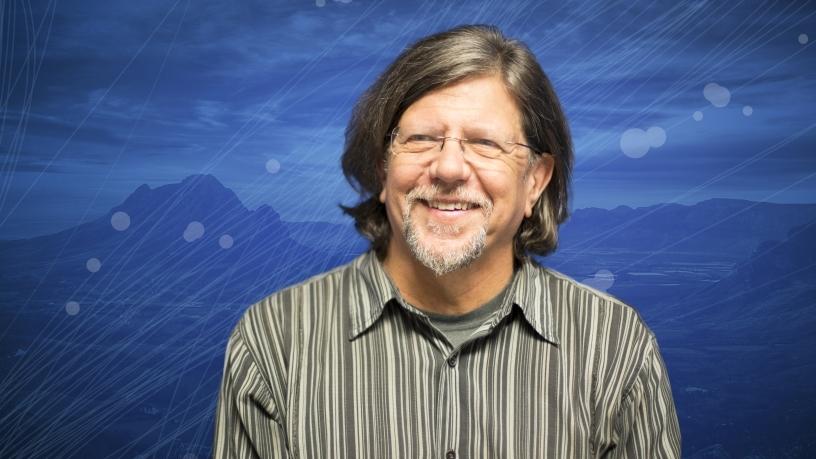
Fast data: high velocity, volume and variety, in real-time. Any business that is invested in supply chain management needs to be able to adapt quickly when the unpredictable happens, and this means having ways to effectively use its data, says Ian de Beer, CEO and founder of zenAptix.
The latest business-use cases of data analytics focus on ways to extract more value from data to streamline business operations, and reduce wastage, unnecessary downtime and hard costs.
Often known as the intelligent application of analytics, businesses are streaming big data and applying predictive modelling and machine learning to adjust to unpredictable events that can cost them significantly.
Fortunately, innovative big data solutions are making it easier to store, and cheaper to extract value from data.
The capability for in-memory storing and in-memory representations of current states of data help manage fast data in real-time; geo-temporal indexing, which relates data to time and space, generates new insights; and improved data visualisations of information and helps end users make more informed decisions about the information they are receiving.
While these approaches are having game-changing effects, the future vision of big data analytics is actually a completely different view of data. It's about monetising data. And in many ways it's already started, as companies employ several ways to create more value from their data.
Sharing data resources to realise more value
Streaming big data means managing high velocity, variety and volume, as well as monitoring of identity driven-entities, like people or vehicles, all of which are uniquely identifiable and are known as trackables.
Each of these 'trackable' units is represented in-memory, and the system ingests any related data from any source, as a streaming real-time data feed.
The result is that the system is able to immediately evaluate any new data relating to the entity, and if there are any rules associated with the change in state with a correlating subscription to the change, a notification is sent out to the parties interested in the state change.
A good example would be a car, belonging to a fleet owned by a company. The car is monitored for speed, excessive acceleration, braking and cornering and whenever these parameters are broken, then the owners of the fleet will be notified. This also takes into account the location of the vehicle and the road on which it is travelling. If the posted speed limit is 60 km/hour and the car is exceeding 80km/hour then that would have an effect on the driver's performance review.
Now take this example into the future with a combination of analytics and exogenous data:
Consider an insurance company wanting to evaluate drivers for an applicable rate for insurance. It is fine to add a unit to the car and assess the driver's behaviour, but this is only a single dimension view. Now if you have access to both location, driving telemetry (braking, acceleration, g-forces, speed) and include weather (visibility, water on the road, cross winds) at that location, time of day, typical traffic density or actual traffic behaviour and other traffic events like traffic lights out, or construction, then the true driving behaviour can be modelled using analytics including multiple, layered data sources.
From a data monetisation perspective the above examples look to multiple sources of data. If this data is able to be ingested into a big data ecosystem then there are many different ways to monetise the data.
In one instance, it could be possible to agree on an abstract value for a particular set of content, such as weather. If a company that specialises in weather data were to partner with another company that also has complementary data, such as dam levels or solar power companies that measure sunlight in areas where the weather company might not, then they can agree on relative value in sharing data.
This is an example of sharing of data resources that allows each company to realise more value within their own domain, but not necessarily reflect a positive impact on the financial books.
A predictive data model to create financial impact
By comparison, a petrochemical company that needs to coordinate deliveries of fuel to its distributors must meet a variety of parameters across its supply chain to ensure the most efficient delivery system. The most ideal situation is when a full tanker is directed to a fuel station that is about to run dry and able to accept a full delivery. However the parameters to meet this situation are specific: The solution requires the accurate evaluation of a prior time-window of fuel levels at each of the distributors.
Additionally, it requires training a predictive model to consider all the features of the fuel data, alongside data relating to price hikes, strike season, weather, long weekends, summer and winter months. Once the predictive model is trained then this can evaluate the streamed data that is being received to be able to direct fuel deliveries with high level of accuracy.
This predictive model in deliveries will ensure the most efficient use of resources, maximise delivery volume, minimise returns and ensure that a run-dry situation does not happen.
In this context, extracting more value out of the data would result in all the distributors streaming current fuel levels back into the system, allowing for intelligence so that a tanker can adjust a delivery to another distributor in the area, and thereby optimising delivery routes. Equally, data relating to price hikes, strike season, weather, long weekends, summer and winter months are all examples of data that may be monetised.
Leveraging data visualisations to make more informed use of data; forecasting against unpredictable events
In the example of a classic SME business, Sandi, in this instance, owns a shop which is one of several spread across the country. By receiving, storing and analysing multiple streams of data in real-time Sandi, the end user, has the information she needs to forecast production and ensure she's constantly meeting her customers' requirements.
The result of all these use-cases is that companies can create actionable predictions out of its data and thereby create a financial impact on their books. The impact of these analytics is further enhanced with shared industry data. No matter which industry: banking, insurance, healthcare, FMCG or petrochemical, the more available industry data, the better the insights.
The future vision of data analytics is to monetise your data
A vision of data analytics of the future does not stop at shared industry insights. It is a world of interoperable data, where companies share industry data to collectively contribute towards faster innovations and customer delivery. In this world, companies that realise the value of interoperable data understand that collectively they all also contribute against inaccuracies, risks, fraud and poor customer experiences.
This is a future where business leaders have completely reframed their thinking around data. Leaving behind the current version of business intelligence, but an interoperable world where companies are able monetise their data.
At this stage in the information age, no company can afford to let their data assets sit idle while their competitors are leveraging off theirs, forging ahead into the 4th Industrial Revolution and the data economy.
Those companies racing to keep ahead of the influx of raw data want to make sense of it in a meaningful way. But having these skills in-house is not always realistic. While there is an argument to say every industry is a technology industry, businesses still have to focus on core competencies to maintain their market position.
A big data analytics team, strategically structured to bring together the best skills in the country, across all analytical functional areas, primed to assist companies with generating meaningful actionable insights, is best placed to assist companies with their digital transformation and be the first to monetise their data for growth.
Share