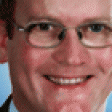
Data quality is a challenge senior executives are often aware of, but fail to do anything about. This is mainly due to a simple fact - it does not rise to the top of their often very long priority lists. Getting the message across to business is becoming increasingly important, as the value of data grows and the cost of poor quality data begins to mount.
A low data error rate of less than 5% can add nearly 30% to hidden and non-value-adding costs. This often relates to the duplicated effort or 'rework' necessary to correct data that was not captured correctly.
However, in order to get attention, the business case needs to be linked to specific and relevant initiatives the company is currently driving.
For example: in one corporate environment, multiple small projects were identified that each showed a lack of consistent customer data as a significant risk and a key dependency. Each had independently allocated a certain amount of time and effort in order to deliver this dependency. When the cost of each of these uncoordinated sub-projects was calculated, it significantly exceeded the budget necessary to support a focused and lean enterprise data quality and data governance capability. These capabilities need to be focused on addressing the data quality needs of each of these projects through a single, focused effort.
The weakest link
By linking these unrelated issues, each of which had been independently identified by the respective project teams, it became very clear there was not only a business case to improve the quality of customer data, but also there were significant hidden costs already allocated to this. This visibility made the business decision to invest in data quality a simple one.
In other environments, data quality issues may be recognised, but may seem overwhelming to operations staff. This could be due to the fact that they may not have the capacity to address a significant rework, while maintaining existing, 'business as usual' administrative processes. A focus on the most important data is necessary to cut any data quality effort down to a manageable size. This allows an organisation to achieve results quickly.
A smart approach is to apply the 80/20 rule. This approach indentifies the 20% of data that delivers 80% of the business value. Companies need to use this approach and clean it up.
A pragmatic approach to prioritising data records is presented in the Data Excellence Approach, pioneered by Dr Walid el Abed. This is based on three tenets, which include:
- Business value;
- Clear accountability and responsibility for addressing data issues; and
- A metrics-driven approach to calculate the value of each failing record and manage both the remediation process and root cause analysis.
Measuring the value of each failing record allows organisations to fix the most important records first. This reduces the remediation efforts to a manageable number. The measure of how many records captured correctly on the first attempt or 'first time right' is a key metric embedded in this approach.
Measure for measure
A trusted measure of the costs and lost opportunities attributable to poor data quality is critical to getting the support of business. The real benefit that comes with cleaning up business data is the opportunity it represents to gain greater insight into business, improve decision-making, enhance customer and supplier relationships, enhance partner relationships and internal relationships, and most importantly, increase revenue.
Ultimately, the case can be made for an enterprise data quality function to support and co-ordinate the data quality needs of individual tactical projects and to meet strategic business goals. A data quality centre of excellence helps to ensure re-use of data quality processes. For example, by embedding the same validations in common application interfaces, as well as in the extract, transform, load processes used to load external batch data sources.
For most organisations, data capture and modification will be split across multiple systems, from legacy applications on the mainframe, to the client server enterprise resource planning, to cloud-based customer relationship management or other specialist systems. The data quality centre of excellence should be driven by the enterprise data governance function to both monitor the compliance of data to enterprise needs, and to ensure required data standards are applied consistently across all platforms through a "develop once, deploy anywhere" architecture.
Data governance, ultimately, should co-ordinate data quality functions to ensure alignment to business drivers, maximise re-use of data quality processes and manage remediation and root cause analysis. Governance efforts should be measured by the amount a company can reduce due to the impact of poor quality data on the business.
Share